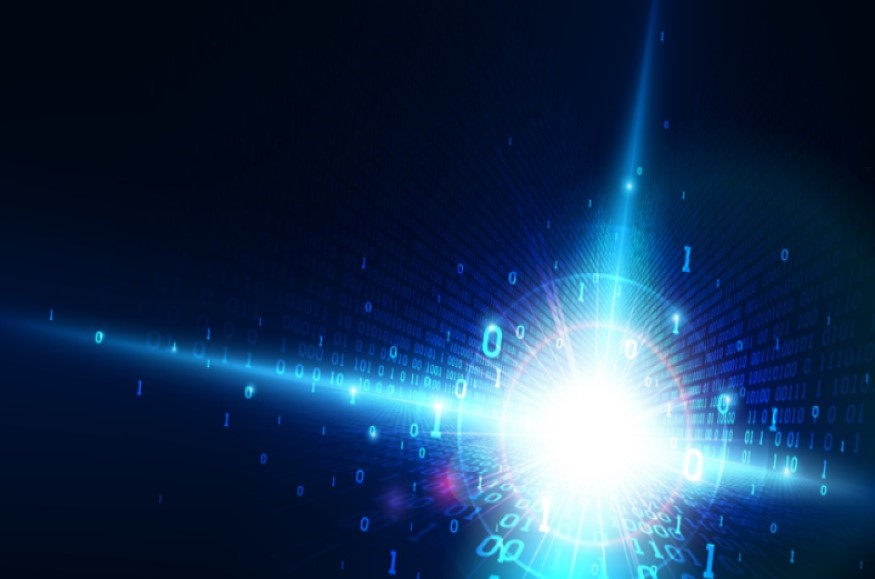
How Are Healthcare AI Developers Responding to WHO’s New Guidance on LLMs?
Med City News
This month, the World Health Organization released new guidelines on the ethics and governance of large language models (LLMs) in healthcare. Reactions from the leaders of healthcare AI companies have been mainly positive.
In its guidance, WHO outlined five broad applications for LLMS in healthcare: diagnosis and clinical care, administrative tasks, education, drug research and development, and patient-guided learning.
While LLMs have potential to improve the state of global healthcare by doing things like alleviating clinical burnout or speeding up drug research, people often have a tendency to “overstate and overestimate” the capabilities of AI, WHO wrote. This can lead to the use of “unproven products” that haven’t been subjected to rigorous evaluation for safety and efficacy, the organization added.
Part of the reason for this is “technological solutionism,” a mindset embodied by those who consider AI tools to be magic bullets capable of eliminating deep social, economic or structural barriers, the guidance stated.
The guidelines stipulated that LLMs intended for healthcare should not be designed only by scientists and engineers — other stakeholders should be included too, such as healthcare providers, patients and clinical researchers. AI developers should give these healthcare stakeholders opportunities to voice concerns and provide input, the guidelines added.
WHO also recommended that healthcare AI companies design LLMs to perform well-defined tasks that improve patient outcomes and boost efficiency for providers — adding that developers should be able to predict and understand any possible secondary outcomes.
Additionally, the guidance stated that AI developers must ensure their product design is inclusive and transparent. This is to ensure LMMs aren’t trained on biased data, whether it’s biased by race, ethnicity, ancestry, sex, gender identity or age.
Leaders from healthcare AI companies have reacted positively to the new guidelines. For instance, Piotr Orzechowski — CEO of Infermedica, a healthcare AI company working to improve preliminary symptom analysis and digital triage — called WHO’s guidance “a significant step” toward ensuring the responsible use of AI in healthcare settings.
“It advocates for global collaboration and strong regulation in the AI healthcare sector, suggesting the creation of a regulatory body similar to those for medical devices. This approach not only ensures patient safety but also recognizes the potential of AI in improving diagnosis and clinical care,” he remarked.
Orzechowsk added that the guidance balances the need for technological advancement with the importance of maintaining the provider-patient relationship.
Jay Anders, chief medical officer at healthcare software company Medicomp Systems, also praised the rules, saying that all healthcare AI needs external regulation.
“[LLMs] need to demonstrate accuracy and consistency in their responses before ever being placed between clinician and patient,” Anders declared.
Another healthcare executive — Michael Gao, CEO and co-founder of SmarterDx, an AI company that provides clinical review and quality audit of medical claims — noted that while the guidelines were correct in stating that hallucinations or inaccurate outputs are among the major risks of LMMs, fear of these risks shouldn’t hinder innovation.
“It’s clear that more work must be done to minimize their impact before AI can be confidently deployed in clinical settings. But a far greater risk is inaction in the face of soaring healthcare costs, which impact both the ability of hospitals to serve their communities and the ability of patients to afford care,” he explained.
Furthermore, an exec from synthetic data company MDClone pointed out that WHO’s guidance may have missed a major topic.
Luz Eruz, MDClone’s chief technology officer, said he welcomes the new guidelines but noticed the guidelines don’t mention synthetic data — non-reversible, artificially created data that replicates the statistical characteristics and correlations of real-world, raw data.
“By combining synthetic data with LLMs, researchers gain the ability to quickly parse and summarize vast amounts of patient data without privacy issues. As a result of these advantages, we expect massive growth in this area, which will present challenges for regulators seeking to keep pace,” Eruz stated.