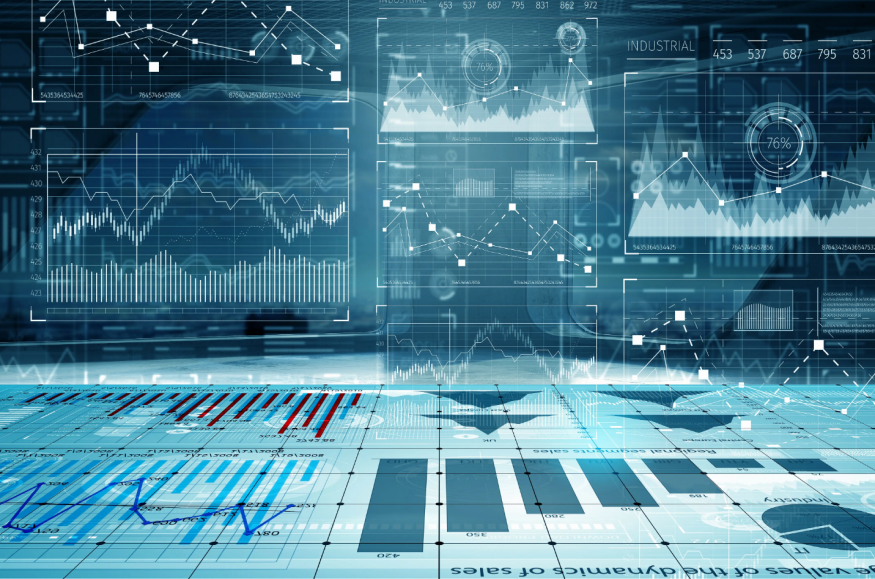
Can Machine Learning be Efficient in Organizing Patient Safety Event Reports?
Applied Clinical Trials Online
Patient safety event reports (PSEs) play an integral role in hospitals for keeping accurate records of adverse events (AEs). A challenge faced by hospitals is efficiently and accurately classifying these AEs due to the sheer number of reports that are created. A recent study published in JMIR turned to machine learning (MI) and artificial intelligence (AI), more specifically language models, to see whether integrating these tools made classification of PSEs more efficient and accurate.
“Recent advancements in text representation, particularly contextual text representation derived from transformer-based language models, offer a promising solution for more precise PSE report classification. Integrating the ML classifier necessitates a balance between human expertise and AI. Central to this integration is the concept of explainability, which is crucial for building trust and ensuring effective human-AI collaboration,” the authors of the study wrote.
The study used a data set of 861 PSEs from a large academic hospital’s maternity units in the Southeastern United States. To classify the reports, various ML classifiers were trained with both static and contextual text representations of PSE reports. The ML classifier’s rationale was derived from a novel explanation technique: the local interpretable model-agnostic explanations (LIME) technique. Finally, an interface that integrates the ML classifier with the LIME technique was designed for the incident reporting system.
The top-performing classifier, which utilized contextual representation, achieved an accuracy of 75.4% while the top performing classifier trained with static text representation was slightly below at 66.7%.
“A PSE reporting interface has been designed to facilitate human-AI collaboration in PSE report classification. In this design, the ML classifier recommends the top 2 most probable event types, along with the explanations for the prediction, enabling PSE reporters and patient safety analysts to choose the most suitable one,” the authors wrote.
In this study, the LIME technique was used to evaluate how the classifier leveraged informative words for classification. In the test data set, 73.8% of reports were categorized into a subset in which at least one highlighted word was deemed relevant to the predicted event type. LIME was able to identify words such as “ibuprofen” and “does” as important words for classifying the report into the medication-related event type. LIME was also able to effectively identify keywords for other event types.
While LIME was able to identify a number of important terms for classifications, the authors concluded that human oversight was still needed as there were some inaccuracies.
“The LIME technique showed that the classifier occasionally relies on arbitrary words for classification, emphasizing the necessity of human oversight,” they wrote.
Overall, the study results show that there is a path to efficiently classifying PSEs with a system that involves both AI and human oversight. Training ML classifiers with contextual text representations can significantly enhance the accuracy of report classification based on the findings, according to the study authors. Further, the authors said they hope that the model designed for this study is just the beginning of more research into efficiently classifying PSEs with AI.
“An event reporting interface that integrates an ML classifier with collaborative decision-making capabilities offers the potential to achieve an efficient and reliable PSE report classification process. These approaches can ultimately help hospitals identify risks and hazards promptly and take timely and informed actions to mitigate adverse events and reduce patient harm,” the authors concluded.