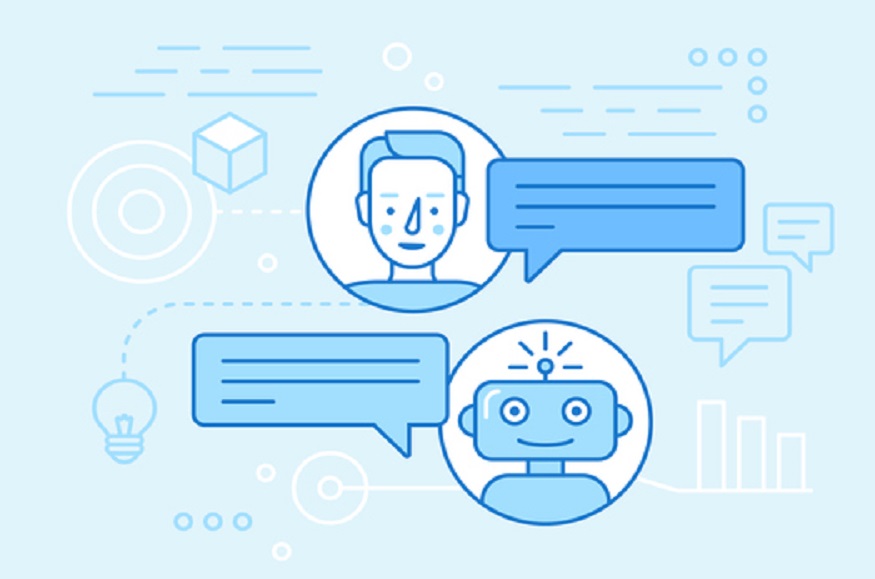
Beyond QA: The Next Wave of Medical Chatbots
Med City News
Medical chatbots have the potential to shape the future of healthcare data retrieval and decision-making support. But this can’t become a reality unless precautions are taken to make sure the answers are both grounded in reality and extracted from reliable sources.
Chatbots have come a long way since inception. While we’ve long used them for shopping and to expedite customer service requests, with growing popularity and use cases, they’re now common in fields from finance to healthcare. In particular, medical chatbots can achieve everything from improving the efficiency and quality of care, to quickly connecting patients to important information or providers.
Like most machine learning tools, the more data a medical chatbot is trained on, the better it will perform. With millions of new biomedical research papers published each year, providing chatbots with fast and reliable answers based on the most current scientific knowledge is paramount. It’s also a Catch 22: the volume of research can quickly outpace the tuning and training of AI models required to keep them accurate.
So how can users stay on top of rapidly evolving medical chatbot capabilities, applications, and benchmarks for success, while also remaining ethical and safe? This article will explore several areas to keep in mind when evaluating the performance of medical chatbots, including credibility, sophistication, and security. Considering chatbots are expected to save businesses up to 2.5 billion hours of work, it’s well worth exploring.
First, let’s consider the parameters medical chatbots should be evaluated on. For the purpose of this article, this includes prebuilt medical knowledge bases such as Pubmed, MedArxiv, and Clinical Trials, user-specific documents, such as internal and confidential files, and structured data in relational databases. With that baseline, we can now dive into the specific areas that contribute to optimal performance of medical chatbots.
Credibility
Before anyone entrusts chatbots in a customer-facing environment, it should first be evaluated for truthfulness, accuracy and explainability. The first, truthfulness, ensures answer fidelity, prioritizing trustworthy sources and avoiding hallucinations. A hallucination is a phenomenon that occurs when ‘eager to please’ AI provides a confident answer that is incorrect. You can see why this would be so detrimental or downright dangerous in a field like medicine.
That’s why it’s so important for medical chatbots to deliver higher accuracy compared to general-purpose large language models (LLMs). LLMs are just a small part of the entire chatbot ecosytem, and people tend to overestimate their abilities. The role of LLMs is to simply digest the information provided by retrieval engines based on knowledge basis. To summarize, it’s the architecture and ecosystem that matters, not the LLM. That’s why explainability is so important. Unlike tools such as ChatGPT, medical chatbots should always cite their sources, so answers are evidence-based. While these tools can do some of the heavy lifting, we’re far from it replacing doctors or humans entirely.
Sophistication
Healthcare is a nuanced industry. Rife with rules, jargon, and best practices that are not widely used or known in other fields, this raises the bar for medical chatbots to succeed. One thing to consider is whether these healthcare-specific AI models take expert preference into consideration. While technologists can get medical chatbots halfway, a team of medical doctors should be consulted to best evaluate the generated answers on relevance, style, consistency, and appropriateness.
Additionally, medical professionals should also help determine if, beyond the generated answers, there exists additional research that can provide a more recent or complete answer. Latency is another important consideration. The speed of building and updating said body of knowledge, calculating embeddings, and running inference to answer user questions is also a determinant to the usefulness of medical chatbots. After all, they’re purpose is to save on time and resources.
Security
Last but not least is security. Beyond regulations unique to the industry—HIPAA, ISO, PCI DSS, etc.—there are several other factors that come into play with medical chatbots. Supporting air-gapped deployment and functioning securely on-premise without requiring internet connectivity or external API calls are two of them. This ensures no sensitive information is shared, and no one has access who shouldn’t.
Another area that should be prioritized is de-identification, which helps discern pertinent information, while redacting personally identifiable information that could compromise patient privacy. In essence, the data that identifies individuals must be stripped out and irreversibly anonymized prior to analysis. In many projects, it’s hard to fully de-identify data, but in the world of healthcare, it’s essential.
Medical chatbots have the potential to shape the future of healthcare data retrieval and decision-making support. But this can’t become a reality unless precautions are taken to make sure the answers are both grounded in reality and extracted from reliable sources. There are few industries in which credibility, sophistication, and security are more important than healthcare. Although perfecting medical chatbots has its challenges, if used properly, the benefits can be game-changing.