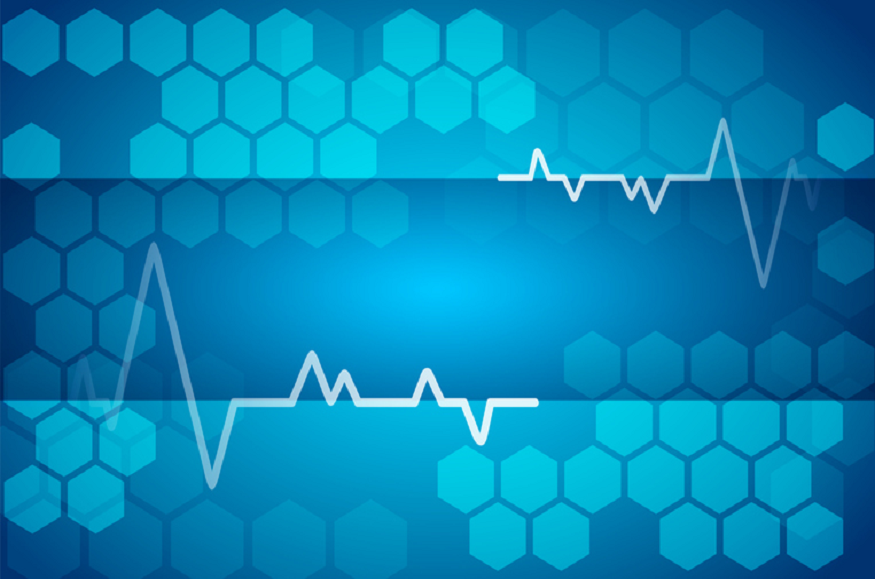
How AI is Accelerating the Need for Real World Data in Healthcare and Life Sciences
MedCity News
Considering the lack of high quality data, companies that excel in compiling and managing data effectively are attracting interest from industry partners and distinguishing themselves from their rivals. Here are some of the ways emerging AI applications are fueling and redefining the need for RWD across HCLS.
The last half decade has brought about change, innovation and overall disruption across healthcare and life sciences (HCLS) that normally transpires over the course of decades. At the forefront of this rapid change has been the ascendency of emerging technologies such as artificial intelligence (AI) and generative artificial intelligence (GenAI). If one takes a step back it is remarkable to reflect on how the discourse surrounding emerging technologies has evolved in just the past two to three years. Conversations that once focused on the ‘what’ now center exclusively on the ‘how’. How can we harness this technology effectively and responsibly? How do we go about upskilling and training medical professionals to use the technology responsibly? How can we ensure we’re maximizing the full capabilities of these tools? Against this backdrop, the rise of these technologies has concurrently shed light to another central need in HCLS: the need for high quality, comprehensive real world data (RWD).
Considering the lack of high quality data, companies that excel in compiling and managing data effectively are attracting interest from industry partners and distinguishing themselves from their rivals.
While there a myriad of factors at play, here are just some of the ways emerging AI applications are fueling and redefining the need for RWD across HCLS.
Elevating healthcare delivery
In many ways, the growth of AI applications and the need for high quality RWD is similar to a relay race. The chain of information clinicians use to diagnose, treat and monitor patients is dependent on the baton of historical data. That data not only needs to be passed effectively but also in a manner that doesn’t overlook critical details. AI presents that critical link in the chain but ultimately the quality of the RWD and the value it can provide is foundational to the success of AI applications.
Reshaping life sciences
Similar to healthcare, AI in life sciences continues to emerge and with it pharmaceutical manufacturers and biotech companies have their own independent initiatives to discover, develop and commercialize therapeutic assets leveraging the technology. Whether seeking out and identifying new therapeutic targets or stratifying patients that are more or less likely to respond to a drug, RWD serves as an anchoring factor. Simply put, biopharma and biotech companies require high quality RWD to power their AI driven innovations.
It begins with data
We’ve heard the old adage that knowledge is power and when it comes to AI applications for healthcare, biopharma and medical device applications, data is that power. In particular authentic, well curated patient data. Yet such data often presents challenges. For example, data might be in silos or exist in different systems. But in order to arrive at clinical grade conclusions, the foundation of such models needs to be patient data. For this reason, the rise of GenAI applications in HCLS makes the need for RWD even greater than existed previously. Gen AI models often produce hallucinations or outcomes that do not exist in the actual data. Such inaccuracies render AI applications unusable, further emphasizing the indispensable role of quality RWD in developing functional and reliable healthcare AI applications.
The path ahead
As applications across HCLS accelerate and in turn the need for RWD to continue to fuel that advancement, the focus will increasingly shift to maximizing value of the data for HCLS organizations. We are already beginning to see healthcare organizations for example that manage patient interactions, ranging from hospitals to home health providers, implementing steps to better organize and prepare their data for downstream uses. This is the case both within their care centers and externally. Investing in data infrastructure, governance processes and strategies to effectively handle RWD is going to be critical for HCLS organizations as they continue on their AI journeys. Those that do so well will be best positioned to derive value from their data while enhancing patient outcomes.