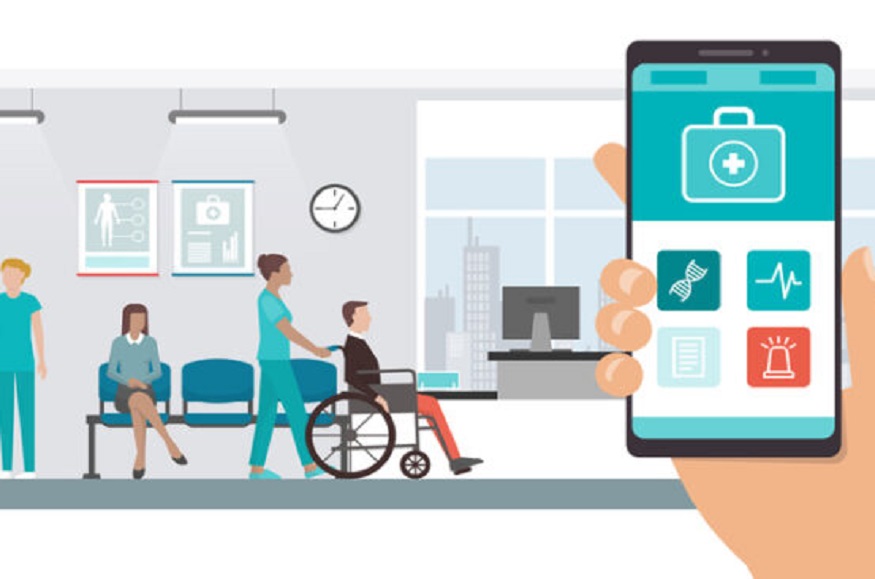
How AI Can Help Hospitals Overcome Patient Flow Barriers
Med City News
Artificial intelligence (AI) holds potential to forecast and predict where hospital leaders should direct limited resources such as clinicians, staff, and beds to improve operational efficiency and drive better patient outcomes.
Although health systems have seen some much-needed improvement to operating margins in recent quarters, these positive headlines mask a disturbing reality that illustrates the immense challenges most health systems still face with hospital operations: 40% of hospitals in the nation are losing money.
For many health systems that are struggling financially, the key step to transforming their hospital operations lies not in hiring more staff or creating more beds. Rather, it comes from utilizing their existing beds more efficiently. In other words, optimizing patient flow.
To optimize patient flow, hospital leaders need new ways of making better, clearer decisions about what is happening at both the macro- and patient-level at each step of the acute care journey from admission to discharge. In this respect, artificial intelligence (AI) holds potential to forecast and predict where hospital leaders should direct limited resources such as clinicians, staff, and beds to improve operational efficiency and drive better patient outcomes.
Four common patient flow challenges
The average adjusted expenses per inpatient day at hospitals was $2,883 in 2021, with a national high of $4,181 in California, according to the Kaiser Family Foundation. Hospitals that underperform their peers relevant to patient avoidable days or length-of-stay (LOS) may do so for a variety of reasons, including staffing issues, geographic challenges, or poor discharge decisions. Regardless of the reason, the result is the same: suboptimal patient flow that compromises patient care, burdens clinicians and staff, and hinders financial performance.
Following are some common obstacles that hospitals frequently face at each stage of the patient flow process:
- Inefficient transfers: For many health systems, the transfer process is associated with a lack of awareness, or slowed, inefficient processes due to extensive manual effort. These problems drive inefficient transfers, which lower the quality of the patient experience, add stress to the workforce, and result in patient leakage as referring facilities and providers select other tertiary care options.
- Variable and delayed discharge planning: Variations and delays in discharge planning leading to excessive avoidable days and ultimately LOS, creating operational inefficiencies for hospitals. When providers and staff lack the necessary tools, capacity, or time to focus on the efficient care progression of each patient, the result is care-progression interruptions and delayed patient discharges.
- Tedious barriers: There are numerous tedious patient flow barriers that come up frequently in the acute patient progression, including diagnostic service delays, consulting provider delays, and care transition delays. These delays can create negative experiences for staff as they have to work around impediments, and patients as they wait for needed care.
- Post-acute care (PAC) access and transportation issues: PAC issues gain greater significance the later they are addressed in the acute care process. If discharge planning begins upon admission and is managed effectively during daily rounds during the inpatient stay, then these roadblocks can be substantially reduced and PAC planning can commence as early as possible in the patient care journey.
Improving patient throughput with AI
Hospitals collect vast amounts of data every day on patient care and operations. However, this data isn’t just useful for retrospective reviews; if enabled with AI technology it can also enable administrators to forecast and prepare for future demand and empower clinicians to make patient flow decisions real-time.
With the emergence of AI, hospitals have a unique opportunity to integrate these data-driven practices into the daily management of patient flow – from admission to discharge. By leveraging AI and predictive modeling, hospitals can uncover relevant patterns and insights in patient flow and care needs from vast amounts of real-time and historical hospital data. These insights are routinely updated based on generic best practices to incorporate recent trends and circumstances to enhance their predictive value, enabling hospitals to manage care more effectively across settings and swiftly adapt to changing circumstances. But if implemented properly, AI can help hospitals create data models that are unique to their business and tailored to their specific operational needs.
One of the most important functions AI can deliver to hospital administrators is to provide clarity on the most relevant issues and metrics that leaders should focus on to accomplish their objectives. For example, if hospital leaders leverage AI to predict available resources based on anticipated patient needs, they can proactively align resources with incoming demand and ensure optimal patient transitions.
Additionally, AI models can be tailored to analyze health system specific patient and hospital data to deliver granular detail to clinicians about the specific operational steps and decisions to take for certain patients. It’s important to note that those steps often differ across health systems, even for similar patient populations.
This approach is a significant departure from the traditional practice of hardwiring best practice alerts into clinicians’ workflows at various critical points. In contrast, AI should sift through each individual health system’s data and models to identify the system wide views as well as unique actions to take for each patient.
For hospital administrators investigating the use of AI, it is critical to first identify which use cases the technology is anticipated to improve. Leaders must avoid the mistake of purchasing an AI solution and then figuring out later which problems to apply it to.
Additionally, when applying AI towards a use case or problem, it is essential that leaders identify some means of changing the process around that use case to extract full value out of the technology investment.
Ultimately, there is no more complicated business in healthcare than the acute-care space. Consequently, adding one piece of technology – no matter how sophisticated the solution – will not make hospitals’ operational challenges disappear. However, by utilizing AI for specific needs that get ahead of issues, deliver the ability to proactively make decisions, and drive operational changes to patient flow, hospital leaders can begin to unlock opportunities to advance patient throughput, operational efficiency, and patient outcomes.